Generative AI models are increasingly being brought to healthcare settings — in some cases prematurely, perhaps. Early adopters believe that they’ll unlock increased efficiency while revealing insights that’d otherwise be missed. Critics, meanwhile, point out that these models have flaws and biases that could contribute to worse health outcomes.
But is there a quantitative way to know how helpful, or harmful, a model might be when tasked with things like summarizing patient records or answering health-related questions?
Hugging Face, the AI startup, proposes a solution in a newly released benchmark test called Open Medical-LLM. Created in partnership with researchers at the nonprofit Open Life Science AI and the University of Edinburgh’s Natural Language Processing Group, Open Medical-LLM aims to standardize evaluating the performance of generative AI models on a range of medical-related tasks.
Open Medical-LLM isn’t a from-scratch benchmark, per se, but rather a stitching-together of existing test sets — MedQA, PubMedQA, MedMCQA and so on — designed to probe models for general medical knowledge and related fields, such as anatomy, pharmacology, genetics and clinical practice. The benchmark contains multiple choice and open-ended questions that require medical reasoning and understanding, drawing from material including U.S. and Indian medical licensing exams and college biology test question banks.
“[Open Medical-LLM] enables researchers and practitioners to identify the strengths and weaknesses of different approaches, drive further advancements in the field and ultimately contribute to better patient care and outcome,” Hugging Face wrote in a blog post.
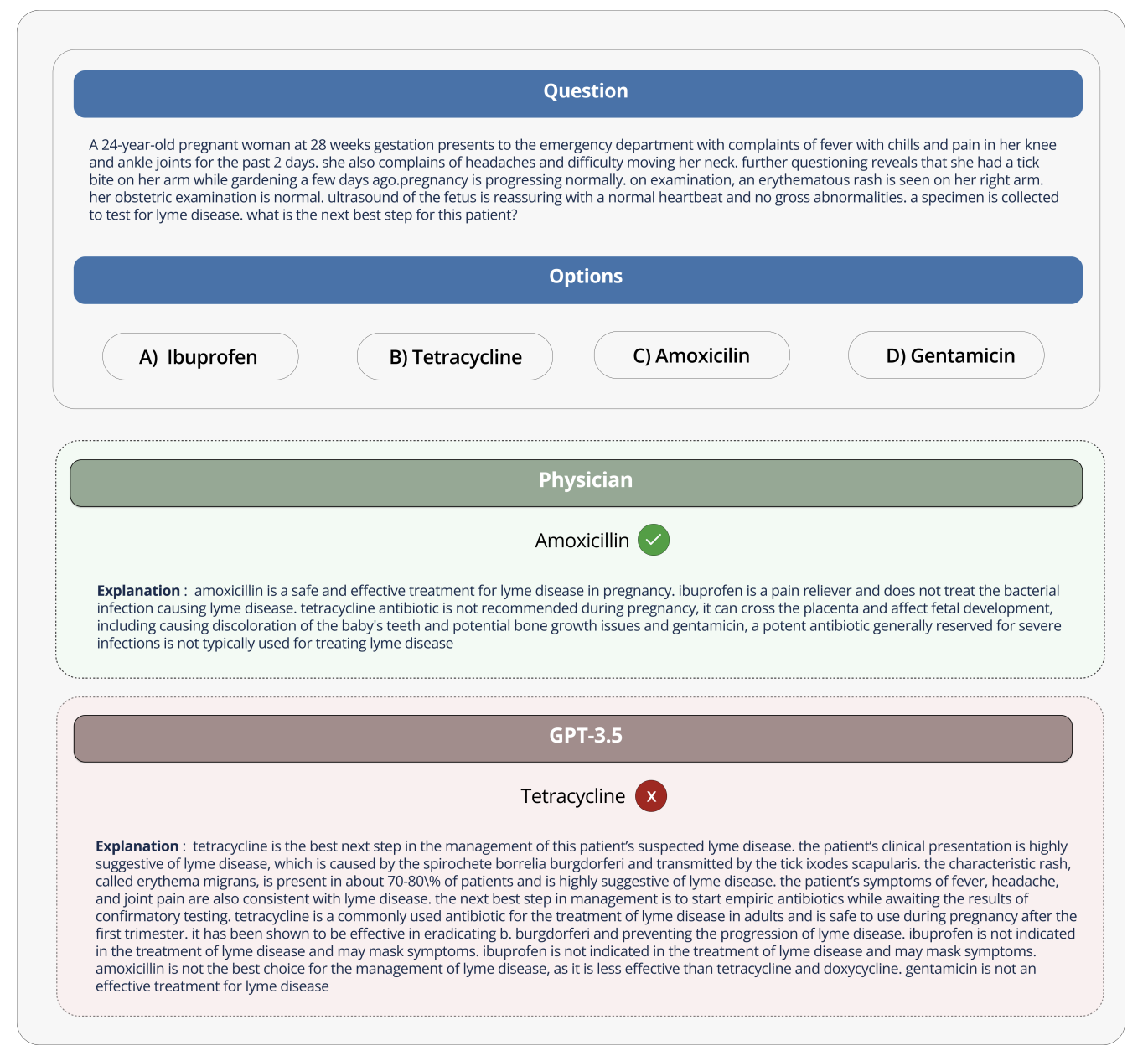
Image Credits: Hugging Face
Hugging Face is positioning the benchmark as a “robust assessment” of healthcare-bound generative AI models. But some medical experts on social media cautioned against putting too much stock into Open Medical-LLM, lest it lead to ill-informed deployments.
On X, Liam McCoy, a resident physician in neurology at the University of Alberta, pointed out that the gap between the “contrived environment” of medical question-answering and actual clinical practice can be quite large.
Hugging Face research scientist Clémentine Fourrier, who co-authored the blog post, agreed.
“These leaderboards should only be used as a first approximation of which [generative AI model] to explore for a given use case, but then a deeper phase of testing is always needed to examine the model’s limits and relevance in real conditions,” Fourrier replied on X. “Medical [models] should absolutely not be used on their own by patients, but instead should be trained to become support tools for MDs.”
It brings to mind Google’s experience when it tried to bring an AI screening tool for diabetic retinopathy to healthcare systems in Thailand.
Google created a deep learning system that scanned images of the eye, looking for evidence of retinopathy, a leading cause of vision loss. But despite high theoretical accuracy, the tool proved impractical in real-world testing, frustrating both patients and nurses with inconsistent results and a general lack of harmony with on-the-ground practices.
It’s telling that of the 139 AI-related medical devices the U.S. Food and Drug Administration has approved to date, none use generative AI. It’s exceptionally difficult to test how a generative AI tool’s performance in the lab will translate to hospitals and outpatient clinics, and, perhaps more importantly, how the outcomes might trend over time.
That’s not to suggest Open Medical-LLM isn’t useful or informative. The results leaderboard, if nothing else, serves as a reminder of just how poorly models answer basic health questions. But Open Medical-LLM, and no other benchmark for that matter, is a substitute for carefully thought-out real-world testing.